In brief
- AI and digitization enable wealth managers to offer tailored automated investment advice.
- Robo-advisors exemplify this trend, using AI to generate insights and recommend suitable investment products.
- Investor reluctance towards AI-driven advice is decreasing, with around 75% of investors comfortable with AI-supported investment advice according to a 2021 Avaloq survey.
Advances in artificial intelligence, and digitization in general, enable wealth managers to provide
automated investment advice tailored to individual client needs. Prominent examples of this trend
are the robo-advisors currently entering the market.
Implemented with a strong partner, AI
creates new insights, generating a holistic picture of the client’s life situation. This helps
wealth managers identify the best matching investment product from the available range.
As
technology advances so investor reluctance decreases, as shown by an Avaloq survey from 2021. In
short, around 75% of investors surveyed said they’d be comfortable with AI-supported or AI-driven
investment advice in the future.
Artificial intelligence
AI and big data are core capabilities for developing modern, user-centered applications. So,
Adaptix Solutions created dedicated AI and data science chapters to bundle expert knowledge and
ensure the
highest-quality delivery.
Figure 1 shows the AI capabilities most important to wealth
management.
As AI’s core capability, machine learning (ML) drove the recent advances in
artificial intelligence. ML enables the training of AI algorithms using large data sets,
ideally, without human intervention. And as such, it relies heavily on the availability of big
data, improving natural language processing (NLP) and robotic process automation (RPA).
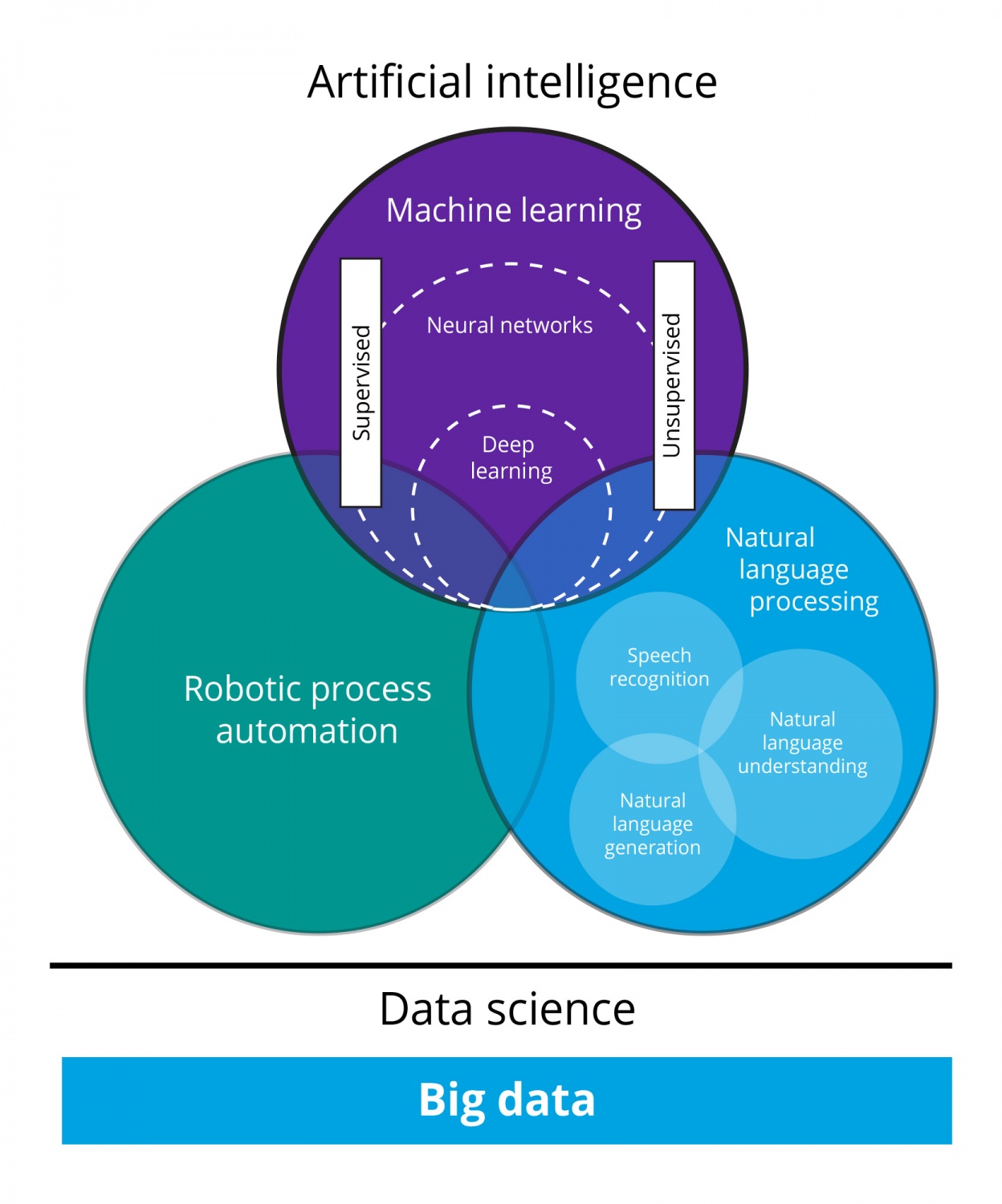
Figure 1: AI in wealth management
Machine learning
Machine learning consists of data-science algorithms and neural networks.
The latter can range from a simple network with a couple neurons to a
sophisticated, multilayered, deep-learning setup.
Depending on the type
of training data, you need to distinguish between supervised and
unsupervised learning.
Supervised learning relies on structured data and
is mainly used for classification; two examples being the risk
classification of stocks, and regression where the house price is a function
of size.
Unsupervised learning can handle unlabeled and unstructured data
like key-value pairs, log files or images. Its best use is for clustering
data or identifying associations. Prominent examples of these are automated
segmentation of the client base, or the identification of certain patterns
in client activities, which could indicate a cross-selling opportunity.
Natural language processing
Natural language processing combines machine learning with linguistic models,
enabling a computer to translate spoken language into text, understand its substance
and generate meaningful text. It uses rule-based and ML approaches to perform
information extraction, named entity recognition and sentiment analysis.
NLP
benefits from recent improvements in deep-learning techniques, pushing chatbots and
virtual assistants to the next level. Shifting from recurrent neural networks (RNN)
to the transformer model, eliminated the sequential structure of RNNs and allowed
for parallelization.
A famous example is the Generative Pre-trained Transformer 3
(GPT-3) created by OpenAI. GPT-3 was trained on hundreds of billions of words and
based on 175 billion ML parameters; it’s the largest non-sparse model to date. Given
a few key words, it can write an entire email that sounds as if it’s been written by
a human.
Robotic process automation
Robotic process automation aims to automate repetitive tasks and business processes. This
might be copying data from a web page into an Excel file, or a complex process like client
onboarding where RPA orchestrates multiple queries to different databases and potential
interactions with the client.
Coupling robotic process automation with machine learning
allows you to use RPA for processes with limited training data. Here, RPA escalates an issue
to a human operator whenever the confidence of a decision is below a certain threshold. The
result is fed back into the solution so that subsequent cases don’t need escalating. This
ensures that the quality of an automated process will improve over time.
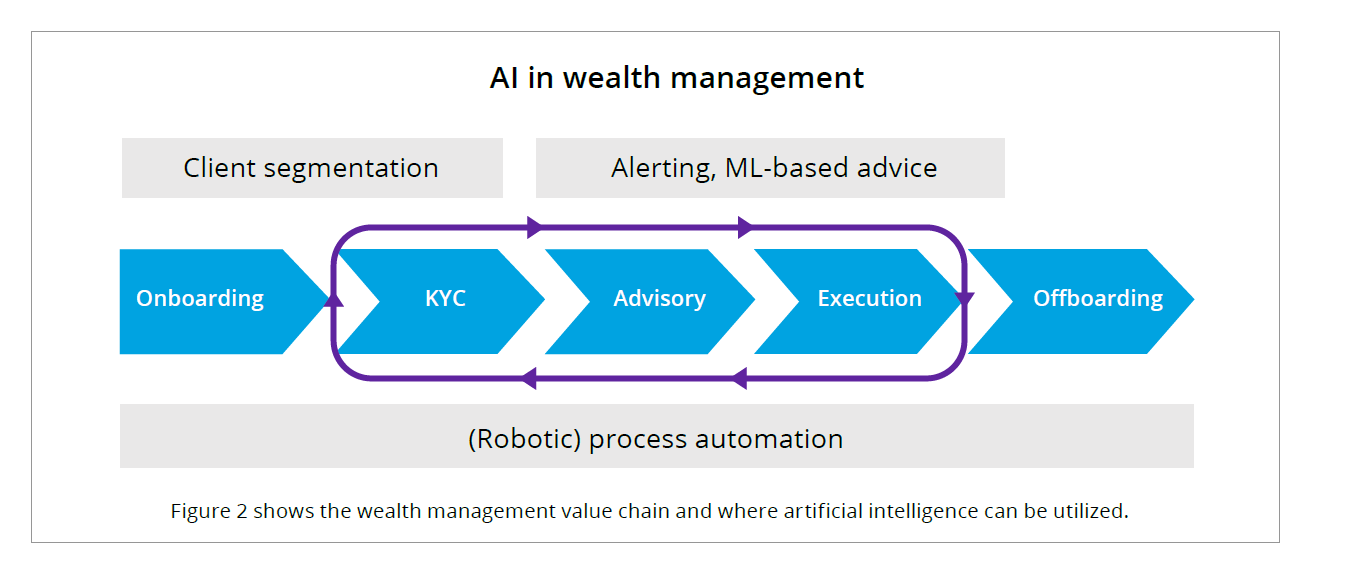
Big data storage
The foundation of a successful AI implementation is the underlying training data. However, when implementing an AI algorithm, you rarely know which data are needed. This uncertainty fueled the popularity of data lakes, which allow data scientists to store structured and unstructured data with ease. When needed, these data can be normalized for supervised learning or used directly for unsupervised learning.
Process automation
As mentioned above, RPA and AI allow the automation of higher-order processes.
A prominent
example is the chatbot which helps potential clients during the onboarding process and the
execution of self-service processes.
Another example is the use of NLP for standard client
interactions. Here, NLP can prewrite emails to answer recurring client requests.
When it
comes to the extraction, validation and digitization of large volumes of data, RPA enables the
automation of error detection and correction. As soon as the RPA algorithm detects an error it
can’t fix by itself, it notifies the appropriate person to fix the error and observes the
remediation. Should this kind of error occur again, the algorithm will be able to fix it
automatically.
Client segmentation
Historically, client segmentation in wealth management was based on simplistic metrics like assets
under management and client profitability. Using ML allows the creation of holistic client models.
In return, this helps wealth managers increase revenue and grow their businesses as they’re able to
identify which clients represent the best growth potential, which clients to maintain and which ones
to disengage from.
For example, analyzing all your client interactions, be they via email, mobile
or messenger, AI algorithms can determine how easy it is to do business with them. This analysis
could include the overall duration of the interaction as well as the style of communication.
Ultimately, this approach allows individual predictions of client growth potential and a fact-based
decision on the effort and time to invest in each client.
More importantly, client segmentation
is not static and AI technologies allow for adjustments in near-real time (should the data show a
significant change in the relationship on your client’s side). Buying a house usually reduces the
client’s risk appetite in the short term.
Monitoring, alerting and cross-selling
Similar to real-time client segmentation, AI technology can be used to monitor client and
marketing activity to create specific alerts and facilitate the cross-selling of financial
products.
For example, previously unnoticed and recurring payments for baby essentials would
raise an alert to discuss fund savings plans for children. On the other hand, past behavior of
leaving clients enables AI algorithms to identify clients with a high churn risk. This allows
relationship managers to conduct a client meeting before he or she decides to leave.
Robo-advisory
While many robo-advisors are based on Markowitz’s Modern Portfolio Theory, the most successful ones rely on AI algorithms.
For example,
NLP can help with sentiment analysis of social media posts and analysis of annual reports or
news sites. Neural networks can be used to predict stock returns and ML, to identify the most
relevant variables for these predictions.
For institutional wealth managers, neural networks
can also help replicate an index by holding a fraction of constituents and reducing portfolio
management costs.
Overall, AI enables tailor-made proposals across all asset classes.
Especially when it comes to securities, ML allows wealth managers to drop the standard
one-size-fits-all approach. It can provide the RM with a selected list of opportunities in line
with the risk appetite and personal situation of the client.
Find a partner to eliminate roadblocks
In 2020, BCG analysis showed that only 10% of companies gained significant financial
benefit from AI. And in 2022, Gartner reported that only 20% of AI projects will have scaled in
enterprises. Failure is mostly driven by a lack of experience in how to educate an organization in
using the technology effectively. It pays to collaborate with an experienced partner like Adaptix
Solutions,
who can either act as an advisor or assume control of the entire project delivery.
Your partner
should also ensure AI projects adhere to the following core principles, which can reduce associated
risks significantly:
- Identify the right business cases. Look for business cases which are based on reusable solutions. For instance, robo advisory builds on AI-based client segmentation
- Educate your organization. AI integration is a joint effort. IT, business and project sponsors must understand the benefits and limitations of AI, and agree on how to measure success. In addition, engage end-users right from the start
- Manage your data and prevent data-driven bias. AI runs on data which should be available in real time, ideally. Know your data sources and implement automated loading or updating processes. Once in place, clean data and test algorithms thoroughly, to avoid data-driven bias
This helps you avoid dead ends, and prevents wasting time and money. With the right partner, you’ll be able to establish a sound basis for AI implementation and rapidly execute adjustments based on real-time, data-driven insights.
Next step
AI has the potential to improve quality and efficiency in wealth management, allowing advisors a
tighter focus on client interaction.
Migration to an AI-based business model requires a
continuous team effort. Ideally, the first step focuses on a hybrid model, with AI improving
existing processes and making educated investment suggestions. Once the process is stable and
advisors are comfortable using the system, full automation toward the client can be gradually
introduced.
So, if individual investment advice is on your strategic road map, and you’re getting
a little concerned about how to actually deliver the business benefits, feel free to contact me. Adaptix Solutions can
help you to
make your AI strategy a reality.
About Adaptix Solutions
Adaptix Solutions is the design, data and development arm of DXC Technology, providing bespoke, end-to-end technology solutions for mission-critical systems, products and services. We help create data-fueled organizations, solving complex operational, technological and strategic challenges. Our passion is building resilient businesses, while generating new business channels and revenue streams, exceptional user experiences and modernized operations at scale.